How to Improve Your Product Recommendation System
For anyone who’s ever shopped on Amazon or streamed any movies on Netflix, the phrases “recommended for you” and “people who bought/watched (the product/movie you’re viewing) also bought/watched (a suggested product/movie)” will sound familiar.
Basically, it’s the algorithm telling you, “hey, we see you're liking this product. Well, here are some even cooler and newer ones for you to try. And it's sometimes shocking how uncannily accurate these suggestions are.
And it's not just Amazon or Netflix. It’s everywhere - eCommerce stores, YouTube, Instagram, and music streaming services. These features are based on the same tool: product recommendation systems.
In a digital marketplace where consumers have ever-diminishing attention spans, data-based suggestions can help businesses to build much-needed competitive advantage. To illustrate, this McKinsey report indicates that 35% of purchases carried out on Amazon can be directly traced to their recommendation systems.
The essence of these systems is to provide customers with personalised recommendations for products or services. The goal is to improve customer satisfaction and loyalty by providing them with offers tailored to their individual needs and preferences.
Now, get your eyes ready to review product recommendation systems as a concept, how they work and how they can be further improved.
What is a product recommendation system?
A product recommendation system (or engine, as some call it) is an AI-enabled algorithm that collects and analyses data on users’ previous interactions to offer them relevant product recommendations - in real time.
User attention is an increasingly scarce commodity. But with data-based models of user habits, membership-based companies can more accurately figure out how to keep their subscribers hooked.
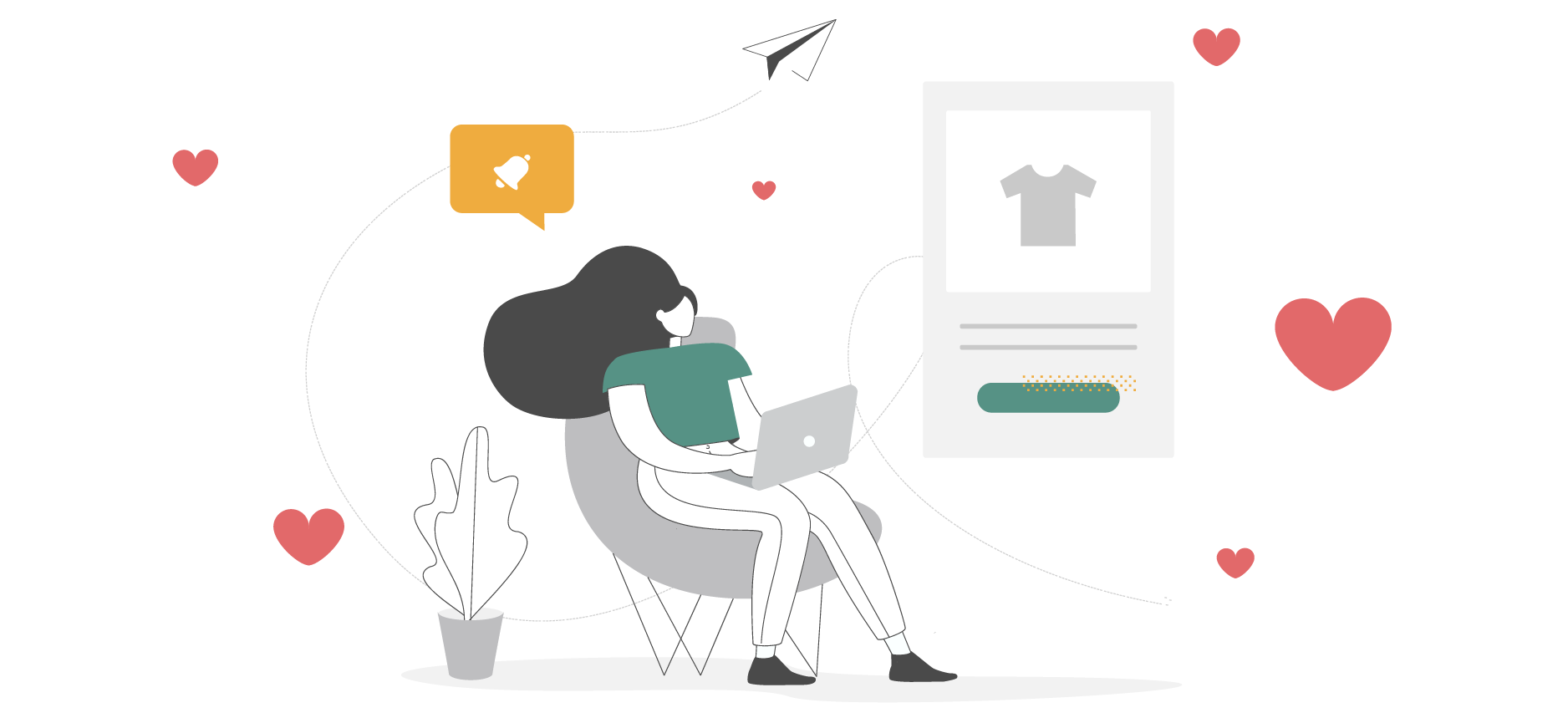
In a world where consumers increasingly prefer personalisation, product recommendation systems enable businesses to provide the ultimate personalised user experience.
For a subscription-based service like Netflix, their product recommendation system contributes tremendously to their engagement numbers. Rather than have subscribers search for titles that might interest them, the algorithm presents them with very similar titles to ones they’ve previously seen and are thus more likely to pique their interest.
And that, there, is the why many Netflix subscribers can’t help but to stay glued to their TV screens.
Types of product recommendation systems
There are three major types of product recommendation systems adopted by businesses - content-based filtering, collaborative filtering, and the last type is a hybrid of both.
Content-based filtering
Content-based filtering is probably the most commonly used type of product recommendation engine. By collecting metadata on a user's previous activity, the algorithm can make recommendations for products that are the most similar to their previous choices.
Thus, if Gunnar has routinely listened to mostly ABBA music albums on Spotify over the past few months, a content-based recommendation system would promptly recommend more ABBA music to him when next he logs in. This framework underpins the “what you might like” sections on Amazon, Netflix, Instagram and Spotify.
Collaborative filtering
Collaborative filtering is another commonly used type of product recommendation system. Its common knowledge that when people have similar personality traits or have bought similar products in the past, they very likely have similar interests.
Thus, If Astrid lives in Sӧdermalm where the residents tend to share an affinity for hip culture, she’ll likely receive more Amazon suggestions recommending the hip wear items most popular among Amazon customers in her neighbourhood because the algorithm expects that she’ll want to dress much like her neighbours.
Of course, it's a bit more complex than that, but that's just a picture of how it works. This is the tool behind the “people who bought (the product you’re viewing) also bought (a suggested product)” and “people in your locations also liked” suggestions.
Companies like Amazon use item-to-item collaborative filtering for most of their web pages and e-mail marketing efforts. It was invented by them in 1998.
Hybrid recommendation system
Both the content-based and collaborative filtering techniques have individual drawbacks. Collaborative filtering, for one, doesn’t work well with new products that have yet to rack up ratings. And content-based filtering, on the other hand, can get over specific, tending to narrow the scope of choices available to users.
The hybrid recommendation system combines the strengths of both techniques to create a more effective model. By weighing users’ tastes against their peers’, the model derives more accurate suggestions.
How to improve your product recommendation system
Product recommendation systems are a key part of many e-commerce platforms, helping shoppers find new and relevant products to buy. A well-designed product recommendation system can provide significant value to both shoppers and businesses, but only if it is constantly updated and refined.
Just like every business tool, companies need to follow certain best practices to get the best returns on their product recommendation systems:
Select the right data sets
As anyone would expect, the lifeblood of an AI-based data analysis tool is the data itself. The accuracy of the algorithm derives from the available data. And while quantity is necessary, it's not as much about how much data you have as it is about its quality.
Data items like transaction history and browser history are far more important for building an accurate recommendation model than survey answers.
The key here is to identify what inputs are essential for the model you’re trying to build and then decide what data sets satisfy that need. Unnecessary data sets (or noise) will only serve as a clutter of data which could confuse or adversely affect the algorithm’s output.
Designate and track appropriate metrics
Regardless of how brilliant you might think an algorithm is, it's only great if it triggers the proper responses from your audience. If you’re to have any chance at improving your recommendation system. you’ll need to develop specific metrics to track its performance so that you can pinpoint any possible pain points and defuse them as fast as possible.
Chief among the metrics that you might want to track is how often users engage with the system’s recommendations. If the number is low, this might mean that the program is not as accurate as you probably want it to be.
Ensure that your model allows variations
Quite often, over-specialization is a trap that product recommendation systems tend to fall into. Here, the model assimilates specific user data sets so routinely that it only recommends the same set of products time after time.
There is only so many World War II documentaries the average viewer can watch before they decide that they’ve had enough. To fix that, Netflix’s algorithm presents users with an array of suggestions, with percentages showing how close they are to their previous interests.
So, rather than see an unlimited WW2 docu-series, the Netflix algorithm may pop up a few marked as a 98% match; and a few other series covering other geopolitical events, marked as 80% matches.
How email recommendation system can be beneficial?
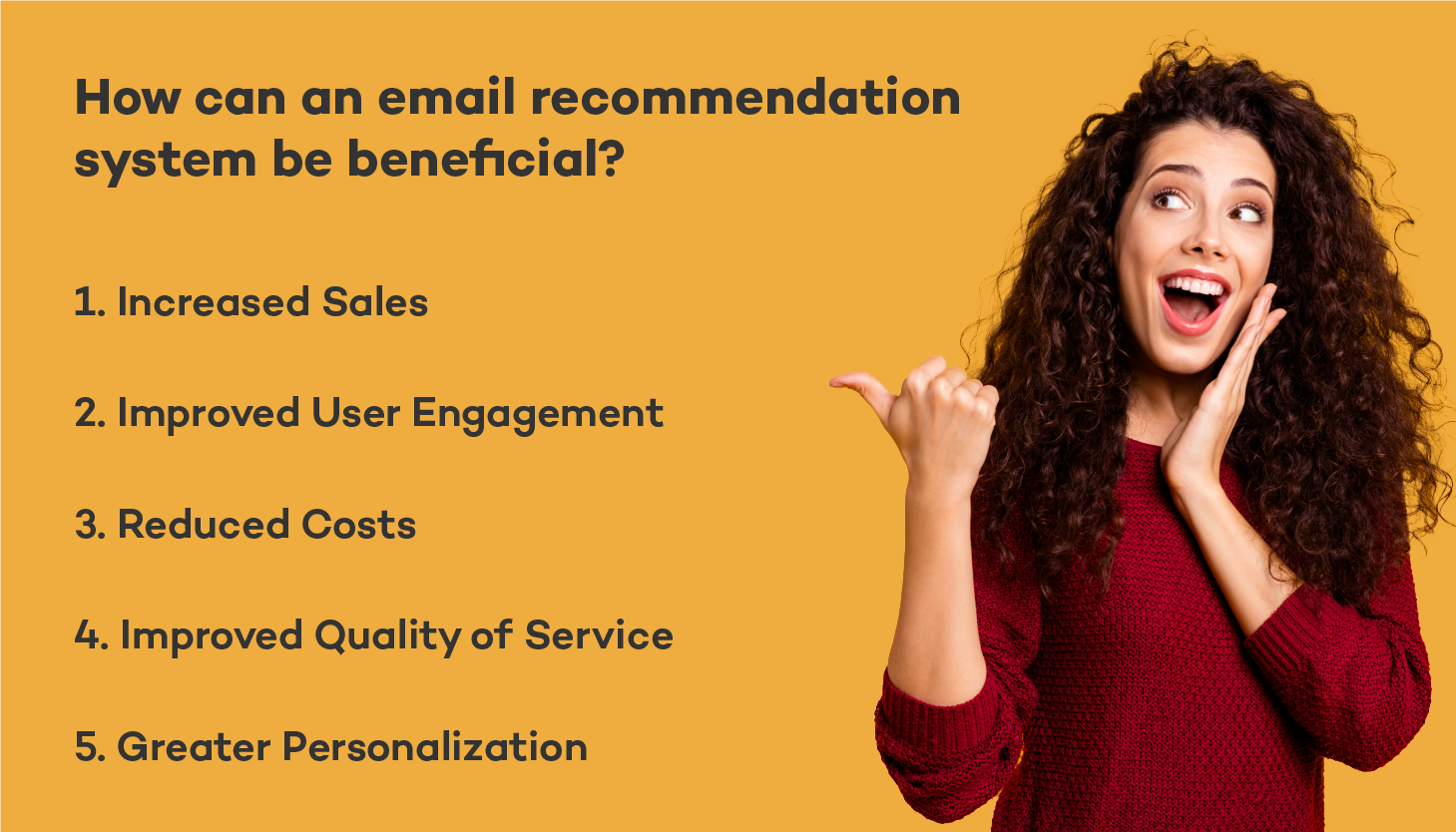
Think of Email recommendation systems as the subtle, personalised variation of aggressive promotional emails.
You can also think of an email recommendation as an invisible shopping assistant, nudging you with an appeasing, tap-on-the-shoulder notification that based on your precious selection, there’re other items you should probably give a try.
Here’re a few benefits of incorporating an email recommendation system into your marketing:
— Increased Sales:
Recommendation systems can increase sales by suggesting items that a user might be interested in. The research is clear: with the ability to boost your click-through rate by 300%+, email recommendation systems remain a sure way to boost engagement levels, and attract traffic into your store, thereby increasing conversion rates.
— Improved User Engagement:
Email Recommendation systems can improve user engagement by suggesting content that a user is likely to find interesting. This can keep subscribers or members engaged with a company's product or service.
— Reduced Costs:
Email Recommendation systems can reduce the costs of customer acquisition and retention. By suggesting items that a subscriber or member is likely to be interested in, recommendation systems can help you save on ad or marketing spend.
— Improved Quality of Service:
Email Recommendation systems can improve the quality of service for your users. By suggesting items, they are likely to be interested in, recommendation systems can help them find the products/services they are looking for.
— Greater Personalisation:
Email Recommendation systems can provide a greater degree of personalization than other types of marketing and advertising. By understanding a user's likes and interests, recommendation systems can suggest items that are tailored to the user's individual taste.
Find out more about tailored email marketing campaigns in our Email Marketing Benchmark report.
Wrap up
By using a product recommendation system to create highly useful suggestions for your consumers, you can improve the quality of your product to gain competitive edge. However, you not only have to abide by best practices, as we’ve highlighted in this article, but you also need to use a compatible solution like Apsis One.
Apsis One provides an entire bouquet of marketing automation capabilities and sundry marketing products to our teeming clientele all over Europe, including businesses in the membership and subscription industries.
With the Apsis One Product Recommendation Add-on, you can create a truly seamless and more accurate product recommendation for your customers — in less time than reading this post did.
Want to see the many other ways we can bring a significant boost customer experience and improve membership engagement? Book a demo for absolutely free today!